Research degrees
PhD opportunities
Visit phd.leeds.ac.uk to search our project ideas, funding opportunities, research areas and PhD supervisors
Search phd.leeds.ac.uk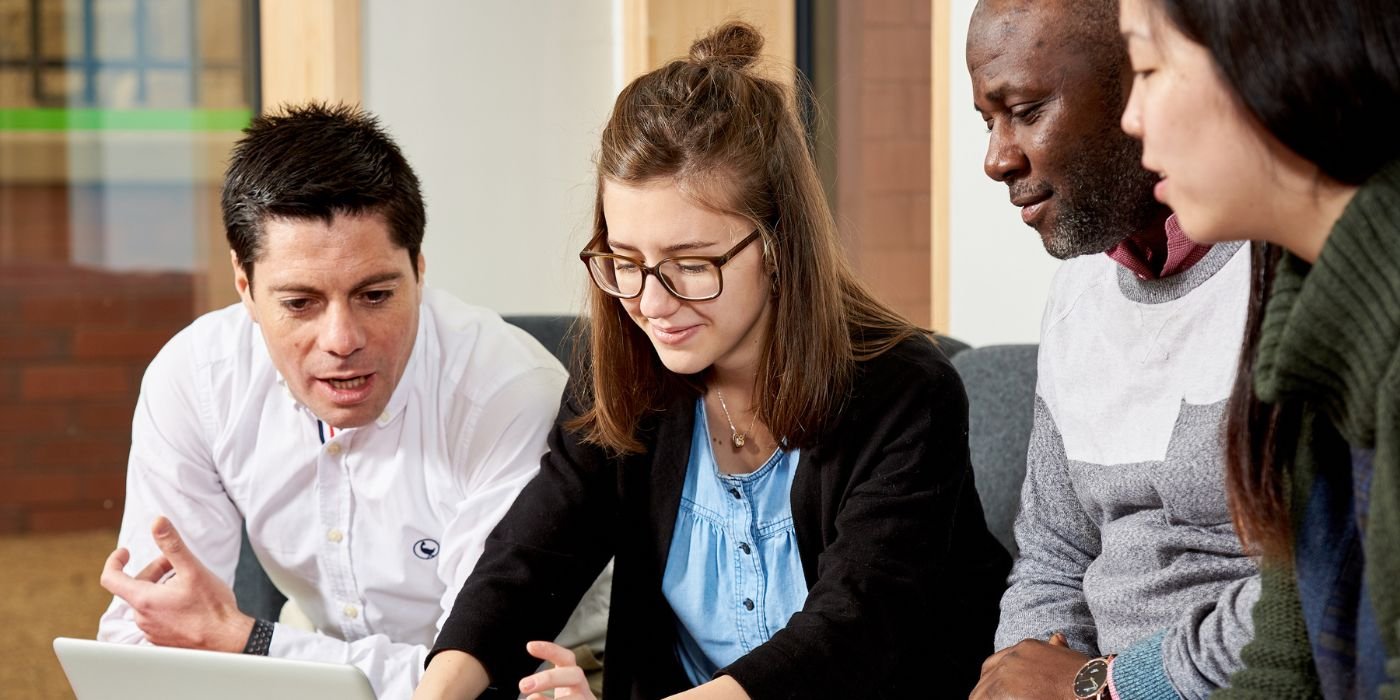
Research degrees
Whether you’re looking to begin an academic career or want to develop your skills and expertise for a career in industry, studying for a PhD will be a journey that will stimulate your passion for your subject and push you to reach your potential.
The Institute for Transport Studies offers three types of research degrees, including a PhD, split-site PhD, and MPhil.
The structure of a typical PhD, usually takes between 3 years full-time or 5 years part-time, during which you’ll be generating new knowledge and considering that new information in relation to existing information. You’ll need to be dedicated and passionate about your area of study. But it’ll be well worth it. Being a doctoral student is challenging, but incredibly rewarding.
Postgraduate research at the Institute for Transport Studies
Why do a research degree at Leeds?
Study in an active research environment: The Institute for Transport is globally renowned for the quality of its research, and the funding it attracts, meaning we’re able to invest in world-class facilities and academic staff who are actively engaged in cutting-edge research. Our research mission is to support the development of intelligent mobility systems that are connected, inclusive, productive and resilient. To find out more, browse a selection of our current and past research projects.
A strong network of support: The Leeds Doctoral College connects our whole researcher community and put you in touch with the services, guidance and opportunities you need. Not only that, you’ll benefit from a strong support network to guide you through your research degree.
Professional skills development: We think of the whole picture at Leeds. That’s why we offer a range of workshops and courses that'll enhance your skillset further and transfer into your professional career.
Close industry links: Our partnerships and links to companies and academic institutions give you the opportunity to network at industry talks, seminars and conferences, building connections that'll benefit your next steps after you complete your PhD.
Global community: You’ll join a friendly, supportive and diverse community of students and researchers who come from all over the world and have access to wellbeing and support services throughout your degree.
Applying for a research degree
How to write a research proposal
Here's how to produce a document that outlines your proposed research topic and programme of research
More on How to write a research proposalHow to apply
Whether you're applying to a project or proposing your own, follow this step-by-step guide to completing your application.
More on How to applyFees
Find out our research degree fees. If you are a former student of the University of Leeds you may be eligible for a 10% alumni tuition fee bursary.
More on FeesEntry requirements
Find out our PhD and MA/MSc Masters by research entry requirements in the Institute for Transport.
More on Entry requirementsVirtuocity
Our world-leading combination of facilities and researchers in a highly innovative, dynamic and multi-disciplinary environment. Virtuocity is a unique programme for city simulation and co-design. It provides an immersive, “human in the loop”, simulation and visualisation facility – “powered” by academic models and industry software, and delivered by an interdisciplinary team of academics with decades of expertise between them.
My PhD experience
Haruko Nakao
Researching with my supervisors makes me feel like we are taking a trip to a new destination. At this point, we don’t always have to know how to get there, but the path always becomes clear.
More on Haruko NakaoDavid Pierce
“Ultimately my research could lead to improved decision-making around transport infrastructure investment which can generate welfare benefits to society.”
More on David PierceAriel Jorre de St Jorre
ITS was a life changing experience for me and it can open a lot of doors for you when applying for jobs.
More on Ariel Jorre de St Jorre